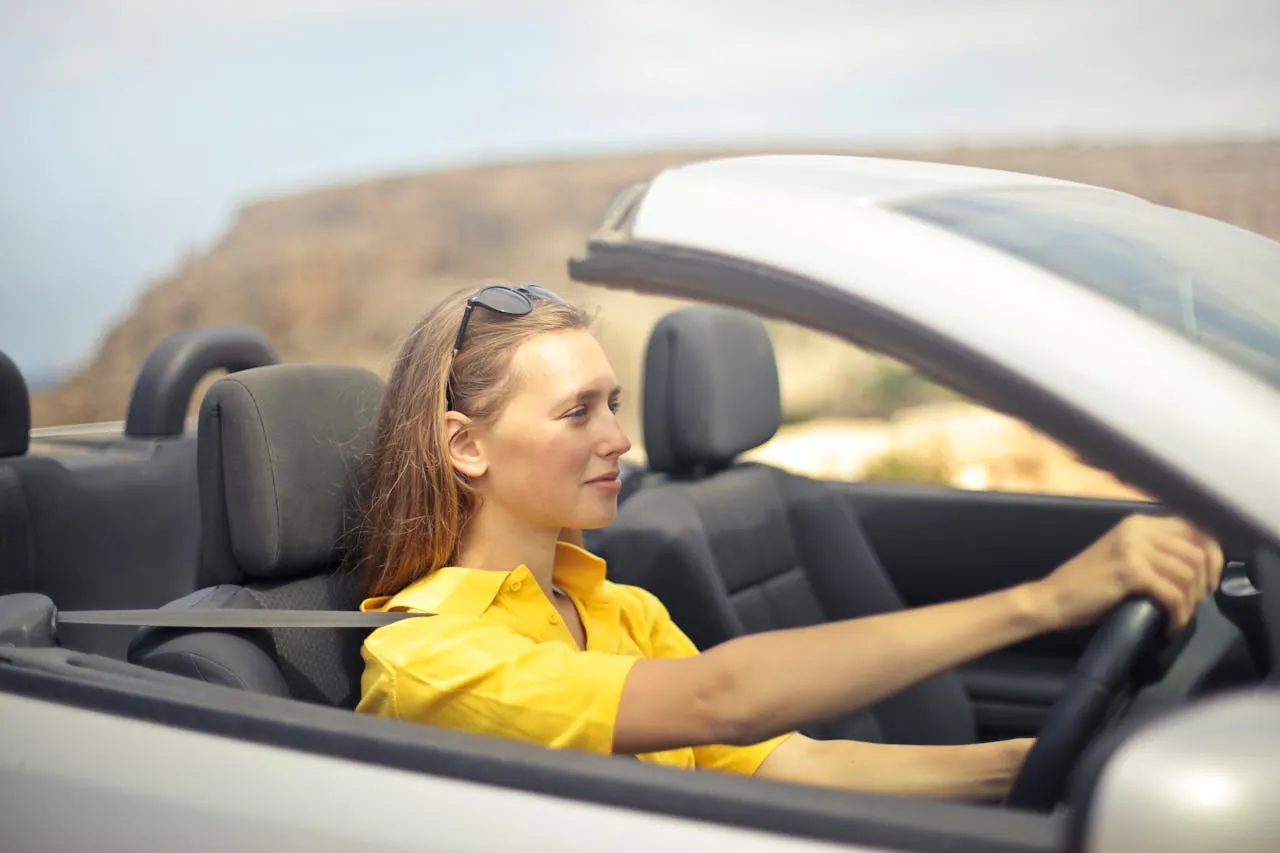
Helm.ai Unveils Vision-Only Path Prediction System for Autonomous Urban Driving
Helm.ai, a pioneering force in advanced artificial intelligence software for high-end Advanced Driver Assistance Systems (ADAS), autonomous driving, and robotics automation, has announced a major breakthrough in real-time path prediction technology. The company introduced Helm.ai Driver, an innovative deep neural network (DNN)-based path prediction system designed specifically for Level 2 through Level 4 autonomous driving in both highway and complex urban environments.
Unlike conventional autonomous vehicle systems that rely on high-definition (HD) maps, lidar, or multiple sensor types to navigate, Helm.ai Driver functions using only vision-based inputs. Leveraging a transformer-based DNN architecture, the system predicts the vehicle’s future path in real time with exceptional precision—completely eliminating the need for pre-mapped environments or costly sensor suites.
At the core of this breakthrough is Helm.ai’s proprietary Deep Teaching™ methodology, a novel approach to neural network training that enables learning directly from large-scale real-world driving data. This technique empowers the model to develop behavior patterns that mimic human driving strategies, particularly in complex urban driving scenarios involving intersections, unprotected turns, dynamic obstacle avoidance, lane changes, and responses to aggressive cut-ins by other vehicles. These behaviors are not hand-coded or rule-based but rather emerge organically from the system’s end-to-end learning framework.
Modular and Scalable Architecture
The Helm.ai Driver integrates seamlessly with the company’s production-grade surround-view perception stack, providing a modular architecture that promotes rapid development, validation efficiency, and cross-system compatibility. By decoupling the perception and path prediction components, Helm.ai enables automotive OEMs and Tier-1 suppliers to plug the solution into existing vehicle platforms without extensive redesign or hardware dependencies.
The company emphasizes that its model’s performance is not only rooted in advanced neural architecture but also in its compatibility with robust perception outputs already validated for real-world driving. This integration allows for enhanced system transparency and interpretability—key factors in building trust with regulators and safety certification bodies.
Real-World Training and Simulation Synergy
To evaluate and refine the capabilities of its path prediction system, Helm.ai conducted extensive closed-loop simulations using the CARLA open-source simulation environment—a popular platform in the autonomous driving research community. In these virtual tests, the Helm.ai Driver module operated within a constantly evolving environment, mirroring the unpredictability and complexity of actual urban traffic.
Augmenting the realism of this simulation was GenSim-2, Helm.ai’s proprietary generative AI foundation model. GenSim-2 enhances the simulation experience by re-rendering synthetic scenes into photorealistic camera outputs. This process ensures that the perception and prediction systems are exposed to high-fidelity visual inputs that closely approximate real-world sensor data, thereby bridging the sim-to-real gap often encountered in autonomous vehicle development.
The closed-loop simulation setup, combining dynamic feedback loops with realistic sensor emulation, allows Helm.ai to validate its path prediction capabilities under a wide range of conditions. This includes not only common urban scenarios but also edge cases and rare events that may be difficult to encounter frequently in naturalistic driving data.
A Leap Toward AI-First Autonomy
“We’re excited to showcase real-time path prediction for urban driving with Helm.ai Driver, based on our proprietary transformer DNN architecture that requires only vision-based perception as input,” said Vladislav Voroninski, CEO and founder of Helm.ai. “By training on real-world data, we developed an advanced path prediction system which mimics the sophisticated behaviors of human drivers, learning end-to-end without any explicitly defined rules.”
Voroninski highlighted the scalability and robustness of the company’s modular AI systems, particularly emphasizing their applicability across various vehicle models, geographic environments, and road conditions. “Importantly, our urban path prediction for L2 through L4 is compatible with our production-grade surround-view vision perception stack. By further validating Helm.ai Driver in a closed-loop simulator, and combining it with our generative AI-based sensor simulation, we’re enabling safer and more scalable development of autonomous driving systems.”
This AI-first approach reflects a broader vision within Helm.ai to redefine the boundaries of autonomous vehicle technology through software innovation rather than hardware dependency. By focusing on vision-only systems trained on massive real-world datasets, the company is positioning itself as a leader in making autonomy more accessible, adaptable, and cost-effective.
From Urban Chaos to Highway Control
One of the standout capabilities of the Helm.ai Driver system is its versatility across different driving domains. While many autonomous solutions require different stacks or tuning for highway versus urban environments, Helm.ai’s model handles both seamlessly.
In urban settings, where unpredictability and dense interactions are the norm, the system demonstrates nuanced decision-making akin to that of a cautious human driver. Whether yielding at unprotected intersections or timing a safe lane merge during heavy traffic, the model reacts in real time using nothing more than visual cues and learned behavioral patterns.
In contrast, on highways where speed and precision take precedence over complex maneuvering, Helm.ai Driver maintains consistent lane keeping, anticipates slower-moving traffic, and adapts fluidly to speed changes and lane closures—all without additional sensors or infrastructure requirements.
The Bigger Picture: A Foundation for Scalable Autonomy
Helm.ai Driver and GenSim-2 are not isolated products but components of Helm.ai’s broader strategy to build an adaptable and scalable software stack for the autonomous vehicle industry. These foundation models are designed to generalize across hardware configurations, making them suitable for diverse automotive applications including passenger vehicles, delivery fleets, and robotics platforms.
By advancing technologies that learn from data—rather than relying on handcrafted rules—Helm.ai is shifting the development paradigm for ADAS and autonomous systems. This shift holds the potential to dramatically reduce development costs, accelerate time-to-market, and enhance long-term system reliability.
, Helm.ai is at the forefront of redefining AI software development for autonomous driving and robotic automation. The company delivers full-stack, real-time AI solutions that include advanced neural networks for both urban and highway driving, as well as development tools and simulation platforms powered by its proprietary Deep Teaching™ methodology and generative AI models.
Collaborating with major global automakers and Tier-1 suppliers, Helm.ai supports production-bound projects aimed at deploying scalable autonomous technology in consumer and commercial vehicles alike. The company continues to expand its product offerings while attracting top engineering talent dedicated to shaping the future of mobility