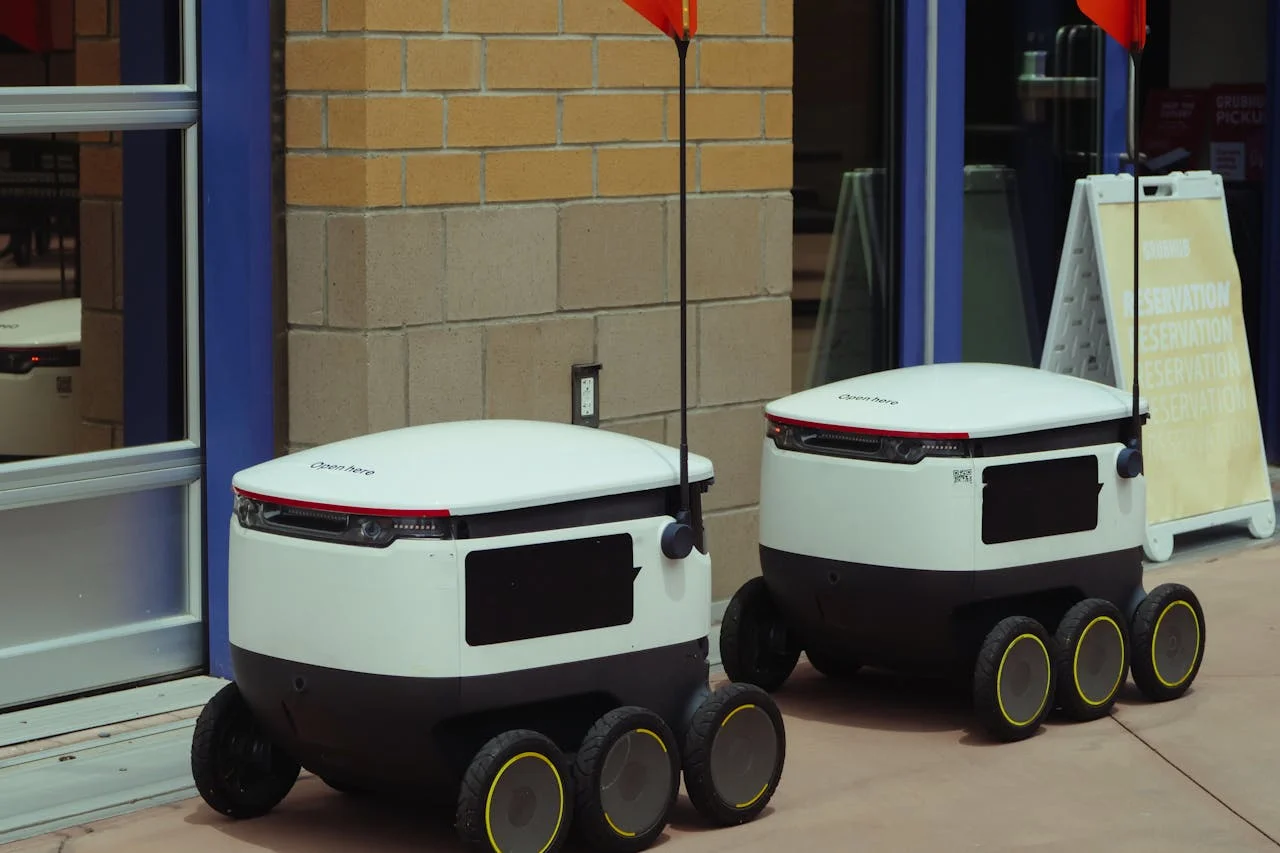
Helm.ai, a leader in AI software for advanced driver assistance systems (ADAS), Level 4 autonomous driving, and robotics, has announced the launch of WorldGen-1, a groundbreaking generative AI model for autonomous vehicles. This multi-sensor base model simulates the entire autonomous driving system, creating highly realistic sensor and perception data across various modalities and perspectives.
WorldGen-1 integrates advanced generative deep neural network (DNN) architectures with deep teaching, a cutting-edge unsupervised training technology. Trained on thousands of hours of diverse driving data, it covers all levels of autonomous driving, including vision, perception, lidar, and odometry.
The model generates realistic sensor data for surround-view cameras, semantic segmentation, lidar front view, lidar bird’s eye view, and the ego vehicle’s path in physical coordinates. By producing comprehensive sensor and path data, WorldGen-1 replicates real-world scenarios from the vehicle’s perspective, facilitating the development and validation of autonomous driving systems.
WorldGen-1 also extrapolates real-world camera data to other modalities, enhancing existing camera datasets with synthetic multi-sensor data. This extension improves dataset richness and reduces data collection costs.
Additionally, WorldGen-1 can predict the behavior of pedestrians, vehicles, and the ego vehicle, generating realistic temporal sequences for up to minutes. This capability supports advanced multi-agent planning and prediction, providing valuable insights for intent prediction and path planning.
Vladislav Voroninski, CEO and co-founder of Helm.ai, stated, “WorldGen-1 bridges the gap between simulation and reality in autonomous driving, offering a scalable and efficient generative AI solution. This innovation accelerates development, enhances safety, and significantly reduces the gap between simulation and real-world testing.”
Voroninski added, “With WorldGen-1, we are creating a vast array of digital representations of real-world driving environments, complete with intelligent agents that simulate human-like prediction and decision-making, helping us address the most complex challenges in autonomous driving.”